Created
April 28, 2021 00:00
-
-
Save luisquintanilla/5c387542240dea57dde3c51521659305 to your computer and use it in GitHub Desktop.
Time Series Forecast Model using ML .NET & Notebooks
This file contains bidirectional Unicode text that may be interpreted or compiled differently than what appears below. To review, open the file in an editor that reveals hidden Unicode characters.
Learn more about bidirectional Unicode characters
{ | |
"cells": [ | |
{ | |
"cell_type": "markdown", | |
"metadata": {}, | |
"source": [ | |
"# Weather forecast model\r\n", | |
"\r\n", | |
"Data is from NOAA. \r\n", | |
"\r\n", | |
"The stations were found using https://www.ncdc.noaa.gov/cdo-web/datatools/findstation\r\n", | |
"\r\n", | |
"The dataset used was the Daily Summaries Dataset -> Air Temperature\r\n", | |
"\r\n", | |
"## Getting the data\r\n", | |
"\r\n", | |
"1. Find a station https://www.ncdc.noaa.gov/cdo-web/datatools/findstation\r\n", | |
"\r\n", | |
"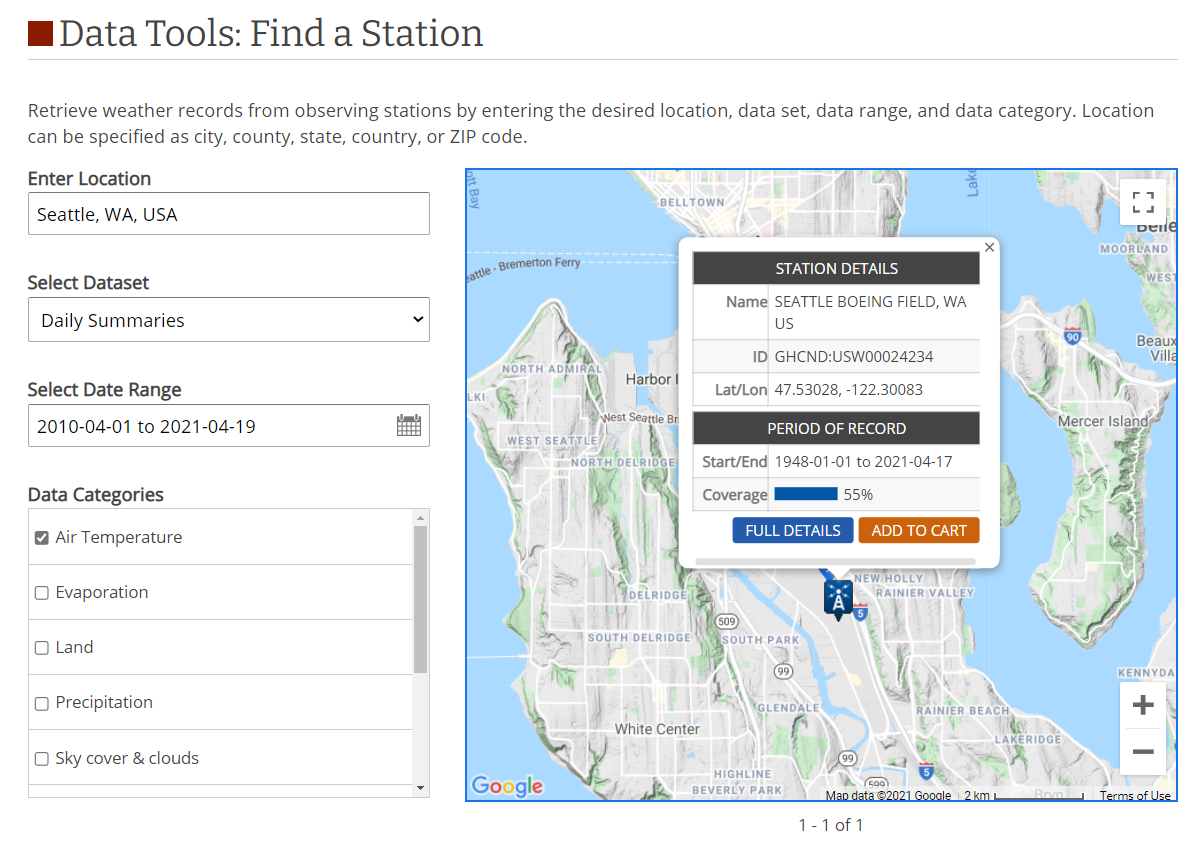\r\n", | |
"\r\n", | |
"1. View cart and export **Custom GHCN-Daily CSV** format\r\n", | |
"\r\n", | |
"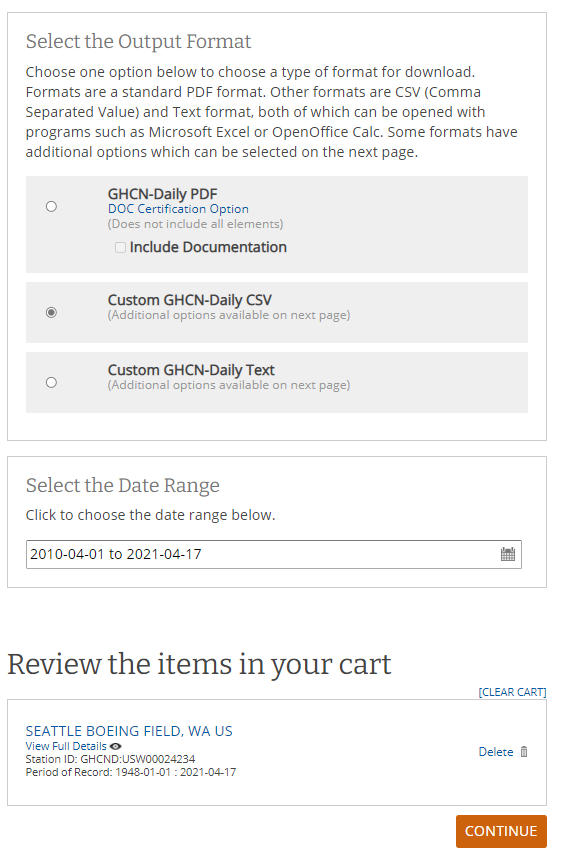\r\n", | |
"\r\n", | |
"1. Submit request for data with the following options:\r\n", | |
" - [x] Station Name\r\n", | |
" - Units: Standard\r\n", | |
" - [x] Air Temperature\r\n", | |
" - [ ] Average Temperature\r\n", | |
" - [x] Maximum Temperature (TMAX)\r\n", | |
" - [x] Minimum Temperature (TMIN)\r\n", | |
"\r\n", | |
"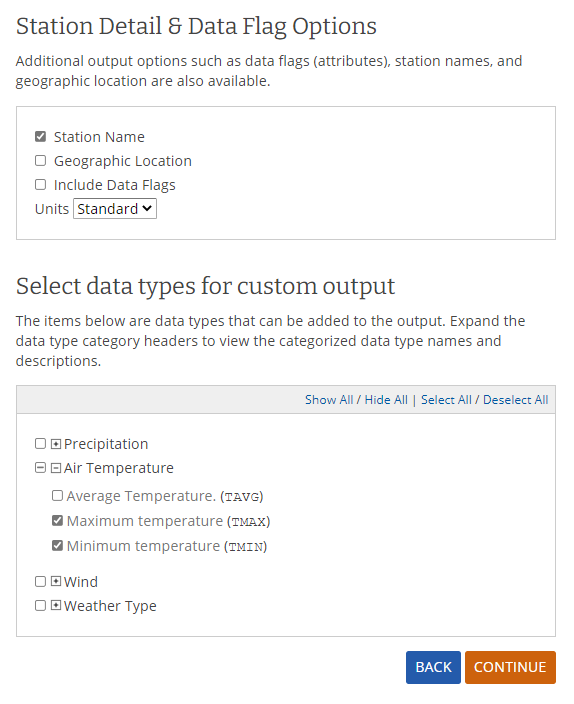" | |
] | |
}, | |
{ | |
"cell_type": "markdown", | |
"metadata": {}, | |
"source": [ | |
"## Install NuGet packages" | |
] | |
}, | |
{ | |
"cell_type": "code", | |
"execution_count": 1, | |
"metadata": { | |
"dotnet_interactive": { | |
"language": "csharp" | |
} | |
}, | |
"outputs": [ | |
{ | |
"data": { | |
"text/html": "\r\n<div>\r\n <div id='dotnet-interactive-this-cell-40348.Microsoft.DotNet.Interactive.Http.HttpPort' style='display: none'>\r\n The below script needs to be able to find the current output cell; this is an easy method to get it.\r\n </div>\r\n <script type='text/javascript'>\r\nasync function probeAddresses(probingAddresses) {\r\n function timeout(ms, promise) {\r\n return new Promise(function (resolve, reject) {\r\n setTimeout(function () {\r\n reject(new Error('timeout'))\r\n }, ms)\r\n promise.then(resolve, reject)\r\n })\r\n }\r\n\r\n if (Array.isArray(probingAddresses)) {\r\n for (let i = 0; i < probingAddresses.length; i++) {\r\n\r\n let rootUrl = probingAddresses[i];\r\n\r\n if (!rootUrl.endsWith('/')) {\r\n rootUrl = `${rootUrl}/`;\r\n }\r\n\r\n try {\r\n let response = await timeout(1000, fetch(`${rootUrl}discovery`, {\r\n method: 'POST',\r\n cache: 'no-cache',\r\n mode: 'cors',\r\n timeout: 1000,\r\n headers: {\r\n 'Content-Type': 'text/plain'\r\n },\r\n body: probingAddresses[i]\r\n }));\r\n\r\n if (response.status == 200) {\r\n return rootUrl;\r\n }\r\n }\r\n catch (e) { }\r\n }\r\n }\r\n}\r\n\r\nfunction loadDotnetInteractiveApi() {\r\n probeAddresses([\"http://172.17.112.1:1000/\", \"http://172.30.64.1:1000/\", \"http://192.168.1.223:1000/\", \"http://100.64.11.55:1000/\", \"http://192.168.1.207:1000/\", \"http://127.0.0.1:1000/\"])\r\n .then((root) => {\r\n // use probing to find host url and api resources\r\n // load interactive helpers and language services\r\n let dotnetInteractiveRequire = require.config({\r\n context: '40348.Microsoft.DotNet.Interactive.Http.HttpPort',\r\n paths:\r\n {\r\n 'dotnet-interactive': `${root}resources`\r\n }\r\n }) || require;\r\n\r\n window.dotnetInteractiveRequire = dotnetInteractiveRequire;\r\n\r\n window.configureRequireFromExtension = function(extensionName, extensionCacheBuster) {\r\n let paths = {};\r\n paths[extensionName] = `${root}extensions/${extensionName}/resources/`;\r\n \r\n let internalRequire = require.config({\r\n context: extensionCacheBuster,\r\n paths: paths,\r\n urlArgs: `cacheBuster=${extensionCacheBuster}`\r\n }) || require;\r\n\r\n return internalRequire\r\n };\r\n \r\n dotnetInteractiveRequire([\r\n 'dotnet-interactive/dotnet-interactive'\r\n ],\r\n function (dotnet) {\r\n dotnet.init(window);\r\n },\r\n function (error) {\r\n console.log(error);\r\n }\r\n );\r\n })\r\n .catch(error => {console.log(error);});\r\n }\r\n\r\n// ensure `require` is available globally\r\nif ((typeof(require) !== typeof(Function)) || (typeof(require.config) !== typeof(Function))) {\r\n let require_script = document.createElement('script');\r\n require_script.setAttribute('src', 'https://cdnjs.cloudflare.com/ajax/libs/require.js/2.3.6/require.min.js');\r\n require_script.setAttribute('type', 'text/javascript');\r\n \r\n \r\n require_script.onload = function() {\r\n loadDotnetInteractiveApi();\r\n };\r\n\r\n document.getElementsByTagName('head')[0].appendChild(require_script);\r\n}\r\nelse {\r\n loadDotnetInteractiveApi();\r\n}\r\n\r\n </script>\r\n</div>" | |
}, | |
"metadata": {}, | |
"output_type": "display_data" | |
}, | |
{ | |
"data": { | |
"text/plain": "Installed package Microsoft.ML.TimeSeries version 1.5.5" | |
}, | |
"metadata": {}, | |
"output_type": "display_data" | |
}, | |
{ | |
"data": { | |
"text/plain": "Installed package Microsoft.ML version 1.5.5" | |
}, | |
"metadata": {}, | |
"output_type": "display_data" | |
} | |
], | |
"source": [ | |
"#r \"nuget:Microsoft.ML,1.5.5\"\r\n", | |
"#r \"nuget:Microsoft.ML.TimeSeries,1.5.5\"" | |
] | |
}, | |
{ | |
"cell_type": "markdown", | |
"metadata": {}, | |
"source": [ | |
"## Import NuGet packages" | |
] | |
}, | |
{ | |
"cell_type": "code", | |
"execution_count": 2, | |
"metadata": {}, | |
"outputs": [], | |
"source": [ | |
"using Microsoft.ML;\r\n", | |
"using Microsoft.ML.Data;\r\n", | |
"using Microsoft.ML.Transforms.TimeSeries;\r\n", | |
"using System.Linq;" | |
] | |
}, | |
{ | |
"cell_type": "markdown", | |
"metadata": {}, | |
"source": [ | |
"## Define model input and output schemas" | |
] | |
}, | |
{ | |
"cell_type": "code", | |
"execution_count": 3, | |
"metadata": { | |
"dotnet_interactive": { | |
"language": "csharp" | |
} | |
}, | |
"outputs": [], | |
"source": [ | |
"public class ModelInput\r\n", | |
"{\r\n", | |
" [LoadColumn(6)]\r\n", | |
" public DateTime Date { get; set; }\r\n", | |
" \r\n", | |
" [LoadColumn(7)]\r\n", | |
" public float MaxTemp { get; set; }\r\n", | |
"\r\n", | |
" [LoadColumn(8)]\r\n", | |
" public float MinTemp {get;set;}\r\n", | |
" \r\n", | |
"}" | |
] | |
}, | |
{ | |
"cell_type": "markdown", | |
"metadata": {}, | |
"source": [ | |
"Added original columns Max / Min Temp columns to compare with actual" | |
] | |
}, | |
{ | |
"cell_type": "code", | |
"execution_count": 4, | |
"metadata": {}, | |
"outputs": [], | |
"source": [ | |
"public class ModelOutput\r\n", | |
"{\r\n", | |
" public DateTime Date { get; set; }\r\n", | |
"\r\n", | |
" public float MaxTemp {get;set;}\r\n", | |
"\r\n", | |
" public float MinTemp {get; set;}\r\n", | |
"\r\n", | |
" public float[] ForecastTemp { get; set; }\r\n", | |
"\r\n", | |
" public float[] LowerBoundTemp { get; set; }\r\n", | |
"\r\n", | |
" public float[] UpperBoundTemp { get; set; }\r\n", | |
"}" | |
] | |
}, | |
{ | |
"cell_type": "markdown", | |
"metadata": {}, | |
"source": [ | |
"## Initialize MLContext" | |
] | |
}, | |
{ | |
"cell_type": "code", | |
"execution_count": 5, | |
"metadata": {}, | |
"outputs": [], | |
"source": [ | |
"var mlContext = new MLContext();" | |
] | |
}, | |
{ | |
"cell_type": "markdown", | |
"metadata": {}, | |
"source": [ | |
"## Load data into IDataView\r\n", | |
"\r\n", | |
"5 year data starts 4/1/2015 \r\n", | |
"10 year data starts 4/2/2010" | |
] | |
}, | |
{ | |
"cell_type": "code", | |
"execution_count": 6, | |
"metadata": {}, | |
"outputs": [], | |
"source": [ | |
"var seattle5yr = \"Data/seattle-5yr.csv\";\r\n", | |
"var seattle10yr = \"Data/seattle-10yr.csv\";\r\n", | |
"\r\n", | |
"var trainingDataView5yr = mlContext.Data.LoadFromTextFile<ModelInput>(seattle5yr, hasHeader: true, separatorChar:',');\r\n", | |
"var trainingDataView10yr = mlContext.Data.LoadFromTextFile<ModelInput>(seattle10yr, hasHeader: true, separatorChar:',');" | |
] | |
}, | |
{ | |
"cell_type": "markdown", | |
"metadata": {}, | |
"source": [ | |
"## Models (5 year data)\r\n", | |
"\r\n", | |
"- Minimum Temperature\r\n", | |
"- Maximum Temperature" | |
] | |
}, | |
{ | |
"cell_type": "markdown", | |
"metadata": {}, | |
"source": [ | |
"### Minimum Temperature" | |
] | |
}, | |
{ | |
"cell_type": "code", | |
"execution_count": 29, | |
"metadata": {}, | |
"outputs": [], | |
"source": [ | |
"IEstimator<ITransformer> minTempEstimator5yr = mlContext.Forecasting.ForecastBySsa(\r\n", | |
" outputColumnName: \"ForecastTemp\",\r\n", | |
" inputColumnName: \"MinTemp\",\r\n", | |
" windowSize: 7,\r\n", | |
" seriesLength: 2202,\r\n", | |
" trainSize: 2202,\r\n", | |
" horizon: 7,\r\n", | |
" confidenceLevel: 0.85f,\r\n", | |
" confidenceLowerBoundColumn: \"LowerBoundTemp\",\r\n", | |
" confidenceUpperBoundColumn: \"UpperBoundTemp\");" | |
] | |
}, | |
{ | |
"cell_type": "code", | |
"execution_count": 30, | |
"metadata": {}, | |
"outputs": [], | |
"source": [ | |
"var minTempModel5yr = minTempEstimator5yr.Fit(trainingDataView5yr);" | |
] | |
}, | |
{ | |
"cell_type": "markdown", | |
"metadata": {}, | |
"source": [ | |
"### Maximum Temperature" | |
] | |
}, | |
{ | |
"cell_type": "code", | |
"execution_count": 31, | |
"metadata": {}, | |
"outputs": [], | |
"source": [ | |
"IEstimator<ITransformer> maxTempEstimator5yr = mlContext.Forecasting.ForecastBySsa(\r\n", | |
" outputColumnName: \"ForecastTemp\",\r\n", | |
" inputColumnName: \"MaxTemp\",\r\n", | |
" windowSize: 7,\r\n", | |
" seriesLength: 2202,\r\n", | |
" trainSize: 2202,\r\n", | |
" horizon: 7,\r\n", | |
" confidenceLevel: 0.85f,\r\n", | |
" confidenceLowerBoundColumn: \"LowerBoundTemp\",\r\n", | |
" confidenceUpperBoundColumn: \"UpperBoundTemp\");" | |
] | |
}, | |
{ | |
"cell_type": "code", | |
"execution_count": 32, | |
"metadata": {}, | |
"outputs": [], | |
"source": [ | |
"var maxTempModel5yr = maxTempEstimator5yr.Fit(trainingDataView5yr);" | |
] | |
}, | |
{ | |
"cell_type": "markdown", | |
"metadata": {}, | |
"source": [ | |
"## Test Models (5 year)" | |
] | |
}, | |
{ | |
"cell_type": "code", | |
"execution_count": 33, | |
"metadata": {}, | |
"outputs": [], | |
"source": [ | |
"var testInput = new ModelInput { Date= new DateTime(2020,04,01), MaxTemp = 51, MinTemp = 38};" | |
] | |
}, | |
{ | |
"cell_type": "code", | |
"execution_count": 34, | |
"metadata": {}, | |
"outputs": [], | |
"source": [ | |
"TimeSeriesPredictionEngine<ModelInput, ModelOutput> minForecastEngine5yr = minTempModel5yr.CreateTimeSeriesEngine<ModelInput, ModelOutput>(mlContext);\r\n", | |
"var minPrediction5yr = minForecastEngine5yr.Predict(testInput,horizon:7);" | |
] | |
}, | |
{ | |
"cell_type": "code", | |
"execution_count": 35, | |
"metadata": {}, | |
"outputs": [], | |
"source": [ | |
"TimeSeriesPredictionEngine<ModelInput, ModelOutput> maxForecastEngine5yr = maxTempModel5yr.CreateTimeSeriesEngine<ModelInput, ModelOutput>(mlContext);\r\n", | |
"var maxPrediction5yr = maxForecastEngine5yr.Predict(testInput, horizon:7);" | |
] | |
}, | |
{ | |
"cell_type": "code", | |
"execution_count": 36, | |
"metadata": { | |
"dotnet_interactive": { | |
"language": "csharp" | |
} | |
}, | |
"outputs": [ | |
{ | |
"data": { | |
"text/html": "<table><thead><tr><th><i>index</i></th><th>Date</th><th>MaxTemp</th><th>MinTemp</th><th>ForecastTemp</th><th>LowerBoundTemp</th><th>UpperBoundTemp</th></tr></thead><tbody><tr><td>0</td><td><span>2020-04-01 00:00:00Z</span></td><td><div class=\"dni-plaintext\">51</div></td><td><div class=\"dni-plaintext\">38</div></td><td><div class=\"dni-plaintext\">[ 45.64229, 45.505856, 45.42607, 45.48796, 45.574562, 45.579506, 45.473053 ]</div></td><td><div class=\"dni-plaintext\">[ 39.421925, 38.72968, 37.953438, 37.29155, 36.686584, 36.047966, 35.26731 ]</div></td><td><div class=\"dni-plaintext\">[ 51.86265, 52.282032, 52.898705, 53.684372, 54.46254, 55.111046, 55.678795 ]</div></td></tr><tr><td>1</td><td><span>2020-04-01 00:00:00Z</span></td><td><div class=\"dni-plaintext\">51</div></td><td><div class=\"dni-plaintext\">38</div></td><td><div class=\"dni-plaintext\">[ 73.780716, 73.05658, 72.64575, 72.69919, 72.88619, 72.93948, 72.638275 ]</div></td><td><div class=\"dni-plaintext\">[ 65.4993, 64.08209, 62.78885, 61.9087, 61.187195, 60.394077, 59.195263 ]</div></td><td><div class=\"dni-plaintext\">[ 82.06213, 82.03107, 82.502655, 83.48968, 84.58519, 85.484886, 86.08128 ]</div></td></tr></tbody></table>" | |
}, | |
"execution_count": 36, | |
"metadata": {}, | |
"output_type": "execute_result" | |
} | |
], | |
"source": [ | |
"new [] {minPrediction5yr, maxPrediction5yr}" | |
] | |
}, | |
{ | |
"cell_type": "markdown", | |
"metadata": {}, | |
"source": [ | |
"## Models (10 year)\r\n", | |
"\r\n", | |
"- Minimum temperature\r\n", | |
"- Maximum temperature" | |
] | |
}, | |
{ | |
"cell_type": "markdown", | |
"metadata": {}, | |
"source": [ | |
"### Minimum Temperature" | |
] | |
}, | |
{ | |
"cell_type": "code", | |
"execution_count": 37, | |
"metadata": {}, | |
"outputs": [], | |
"source": [ | |
"IEstimator<ITransformer> minTempEstimator10yr = mlContext.Forecasting.ForecastBySsa(\r\n", | |
" outputColumnName: \"ForecastTemp\",\r\n", | |
" inputColumnName: \"MinTemp\",\r\n", | |
" windowSize: 7,\r\n", | |
" seriesLength: 4027,\r\n", | |
" trainSize: 4027,\r\n", | |
" horizon: 7,\r\n", | |
" confidenceLevel: 0.85f,\r\n", | |
" confidenceLowerBoundColumn: \"LowerBoundTemp\",\r\n", | |
" confidenceUpperBoundColumn: \"UpperBoundTemp\");" | |
] | |
}, | |
{ | |
"cell_type": "code", | |
"execution_count": 38, | |
"metadata": {}, | |
"outputs": [], | |
"source": [ | |
"var minTempModel10yr = minTempEstimator10yr.Fit(trainingDataView10yr);" | |
] | |
}, | |
{ | |
"cell_type": "markdown", | |
"metadata": {}, | |
"source": [ | |
"### Maximum Temperature" | |
] | |
}, | |
{ | |
"cell_type": "code", | |
"execution_count": 39, | |
"metadata": {}, | |
"outputs": [], | |
"source": [ | |
"IEstimator<ITransformer> maxTempEstimator10yr = mlContext.Forecasting.ForecastBySsa(\r\n", | |
" outputColumnName: \"ForecastTemp\",\r\n", | |
" inputColumnName: \"MaxTemp\",\r\n", | |
" windowSize: 7,\r\n", | |
" seriesLength: 4027,\r\n", | |
" trainSize: 4027,\r\n", | |
" horizon: 7,\r\n", | |
" confidenceLevel: 0.85f,\r\n", | |
" confidenceLowerBoundColumn: \"LowerBoundTemp\",\r\n", | |
" confidenceUpperBoundColumn: \"UpperBoundTemp\");" | |
] | |
}, | |
{ | |
"cell_type": "code", | |
"execution_count": 40, | |
"metadata": {}, | |
"outputs": [], | |
"source": [ | |
"var maxTempModel10yr = maxTempEstimator10yr.Fit(trainingDataView10yr);" | |
] | |
}, | |
{ | |
"cell_type": "markdown", | |
"metadata": {}, | |
"source": [ | |
"## Test Models (10 year)" | |
] | |
}, | |
{ | |
"cell_type": "code", | |
"execution_count": 41, | |
"metadata": {}, | |
"outputs": [], | |
"source": [ | |
"var testInput = new ModelInput { Date= new DateTime(2020,04,01), MaxTemp = 51, MinTemp = 38};" | |
] | |
}, | |
{ | |
"cell_type": "code", | |
"execution_count": 42, | |
"metadata": {}, | |
"outputs": [], | |
"source": [ | |
"TimeSeriesPredictionEngine<ModelInput, ModelOutput> minForecastEngine10yr = minTempModel10yr.CreateTimeSeriesEngine<ModelInput, ModelOutput>(mlContext);\r\n", | |
"var minPrediction10yr = minForecastEngine10yr.Predict(testInput,horizon:7);" | |
] | |
}, | |
{ | |
"cell_type": "code", | |
"execution_count": 43, | |
"metadata": {}, | |
"outputs": [], | |
"source": [ | |
"TimeSeriesPredictionEngine<ModelInput, ModelOutput> maxForecastEngine10yr = maxTempModel10yr.CreateTimeSeriesEngine<ModelInput, ModelOutput>(mlContext);\r\n", | |
"var maxPrediction10yr = maxForecastEngine10yr.Predict(testInput, horizon:7);" | |
] | |
}, | |
{ | |
"cell_type": "code", | |
"execution_count": 44, | |
"metadata": {}, | |
"outputs": [ | |
{ | |
"data": { | |
"text/html": "<table><thead><tr><th><i>index</i></th><th>Date</th><th>MaxTemp</th><th>MinTemp</th><th>ForecastTemp</th><th>LowerBoundTemp</th><th>UpperBoundTemp</th></tr></thead><tbody><tr><td>0</td><td><span>2020-04-01 00:00:00Z</span></td><td><div class=\"dni-plaintext\">51</div></td><td><div class=\"dni-plaintext\">38</div></td><td><div class=\"dni-plaintext\">[ 45.442406, 45.264603, 45.15645, 45.19384, 45.254196, 45.226856, 45.13159 ]</div></td><td><div class=\"dni-plaintext\">[ 39.717957, 39.014584, 38.26309, 37.649364, 37.10386, 36.508354, 35.83022 ]</div></td><td><div class=\"dni-plaintext\">[ 51.166855, 51.51462, 52.04981, 52.738316, 53.404533, 53.94536, 54.432964 ]</div></td></tr><tr><td>1</td><td><span>2020-04-01 00:00:00Z</span></td><td><div class=\"dni-plaintext\">51</div></td><td><div class=\"dni-plaintext\">38</div></td><td><div class=\"dni-plaintext\">[ 73.52904, 72.769394, 72.34601, 72.38786, 72.55228, 72.53551, 72.297585 ]</div></td><td><div class=\"dni-plaintext\">[ 66.13588, 64.76329, 63.576366, 62.831036, 62.2506, 61.534508, 60.570606 ]</div></td><td><div class=\"dni-plaintext\">[ 80.922195, 80.7755, 81.11565, 81.944695, 82.85395, 83.53651, 84.02457 ]</div></td></tr></tbody></table>" | |
}, | |
"execution_count": 44, | |
"metadata": {}, | |
"output_type": "execute_result" | |
} | |
], | |
"source": [ | |
"new [] {minPrediction10yr, maxPrediction10yr}" | |
] | |
}, | |
{ | |
"cell_type": "markdown", | |
"metadata": {}, | |
"source": [ | |
"## Conclusion\r\n", | |
"\r\n", | |
"5 year models, because it has more recent data looks to make better predictions. I recommend playing around with hyperparameters" | |
] | |
} | |
], | |
"metadata": { | |
"kernelspec": { | |
"display_name": ".NET (C#)", | |
"language": "C#", | |
"name": ".net-csharp" | |
}, | |
"language_info": { | |
"file_extension": ".cs", | |
"mimetype": "text/x-csharp", | |
"name": "C#", | |
"pygments_lexer": "csharp", | |
"version": "8.0" | |
}, | |
"orig_nbformat": 2 | |
}, | |
"nbformat": 4, | |
"nbformat_minor": 2 | |
} |
Sign up for free
to join this conversation on GitHub.
Already have an account?
Sign in to comment