Created
April 13, 2023 16:34
-
-
Save CookieLau/b489b37c53e921c8bcd661ad3ce61e17 to your computer and use it in GitHub Desktop.
Foreground/Background Segmentation using ADMM algorithm.
This file contains hidden or bidirectional Unicode text that may be interpreted or compiled differently than what appears below. To review, open the file in an editor that reveals hidden Unicode characters.
Learn more about bidirectional Unicode characters
import time | |
import math | |
import numpy as np | |
import pandas as pd | |
import pickle | |
import wandb | |
wandb.init(project="dsa5103-assignment3") | |
# helper functions | |
def nuclear_norm(X): | |
return np.linalg.norm(X, ord='nuc') | |
# or manually sum the singular values, i.e. \Sigma | |
# return np.sum(np.linalg.svd(A)[1]) | |
def l1_norm(X): | |
return np.linalg.norm(X, ord=1) | |
def frobenius_norm(X): | |
return np.linalg.norm(X, ord='fro') | |
X = np.random.randn(100, 100) | |
assert np.isclose(nuclear_norm(np.array(X)), np.sum(np.linalg.svd(X)[1])), \ | |
f"nuclear norm is not correct, should be {np.sum(np.linalg.svd(X)[1])} but got {nuclear_norm(np.array(X))} " | |
# soft thresholding element-wise | |
def soft_thresholding(X, tau): | |
return np.multiply(np.sign(X), np.maximum(np.abs(X) - tau, 0)) | |
# set the random seed | |
np.random.seed(677) | |
MAX_ITER = 200 | |
def reduced_SVD_tuned_sigma(M): | |
m, n = M.shape | |
k = 0 | |
residual = 0 | |
L = np.zeros((m, n), dtype=np.float64) | |
S = np.zeros((m, n), dtype=np.float64) | |
Z = np.zeros((m, n), dtype=np.float64) | |
rho = 1.1 | |
lam = 1/math.sqrt(max(m, n)) | |
tau = 1 | |
# set sigma the inverse of the largest singular value of M | |
sigma = 1/np.linalg.svd(M, full_matrices=False)[1][0] | |
start = time.time() | |
for k in range(MAX_ITER): | |
old_L = L | |
old_S = S | |
# update sigma | |
sigma = sigma * rho | |
# calculate T^k | |
T = M - S - 1/sigma * Z | |
# reduced SVD decomposition on T | |
U, d, V = np.linalg.svd(T, full_matrices=False) | |
# apply soft thresholding | |
gamma = soft_thresholding(d, 1/sigma) | |
# calcluate new L using full SVD value | |
L = U @ np.diag(gamma) @ V | |
# calculate new S | |
S = soft_thresholding(M - L - 1/sigma * Z, lam/sigma) | |
# calculate new Z | |
Z = Z + tau * sigma * (L + S - M) | |
residual = max(frobenius_norm(L - old_L)/(1+frobenius_norm(L)), frobenius_norm(S - old_S)/(1+frobenius_norm(S))) | |
if residual < 1e-4 or k > MAX_ITER: | |
break | |
wandb.log({"residual": residual, "iteration": k}) | |
print(f"number of iterations: {k}, the break residual is {residual}") | |
end = time.time() | |
return L, S, k, residual, end-start | |
raw_data = pd.read_csv("./BasketballPlayer.csv", header=None) | |
m, n = raw_data.shape | |
#L, S, k, residual, time_cost = reduced_SVD_tuned_sigma(raw_data) | |
L, S, k, residual, time_cost = reduced_SVD_tuned_sigma(raw_data) | |
# save result to pickle files | |
with open("L.pkl", "wb") as f: | |
pickle.dump(L, f) | |
with open("S.pkl", "wb") as f: | |
pickle.dump(S, f) | |
print(f"number of iterations: {k}, the break residual is {residual}, time cost: {time_cost} seconds") | |
Sign up for free
to join this conversation on GitHub.
Already have an account?
Sign in to comment
The foreground is stable:
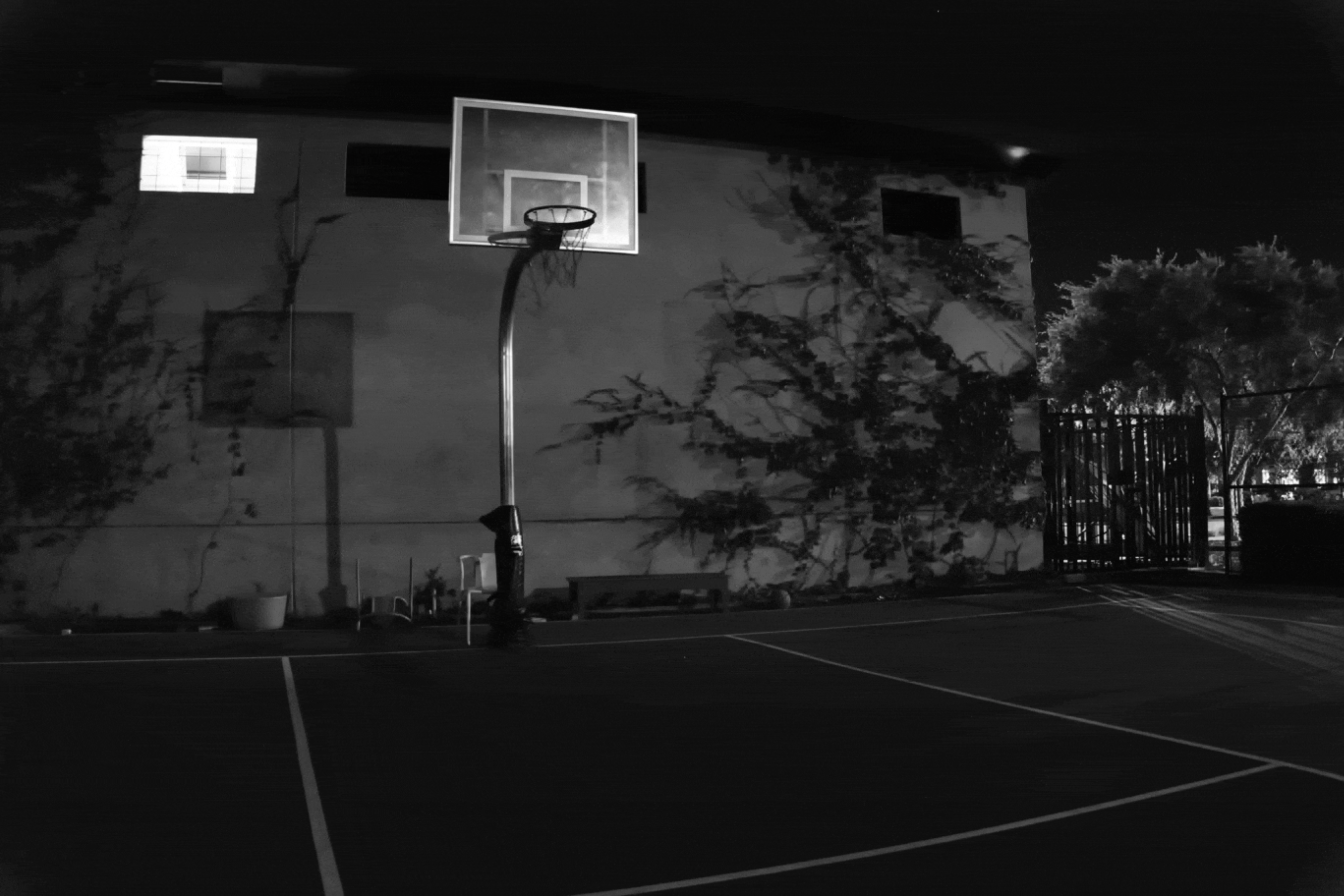
The moving object is:
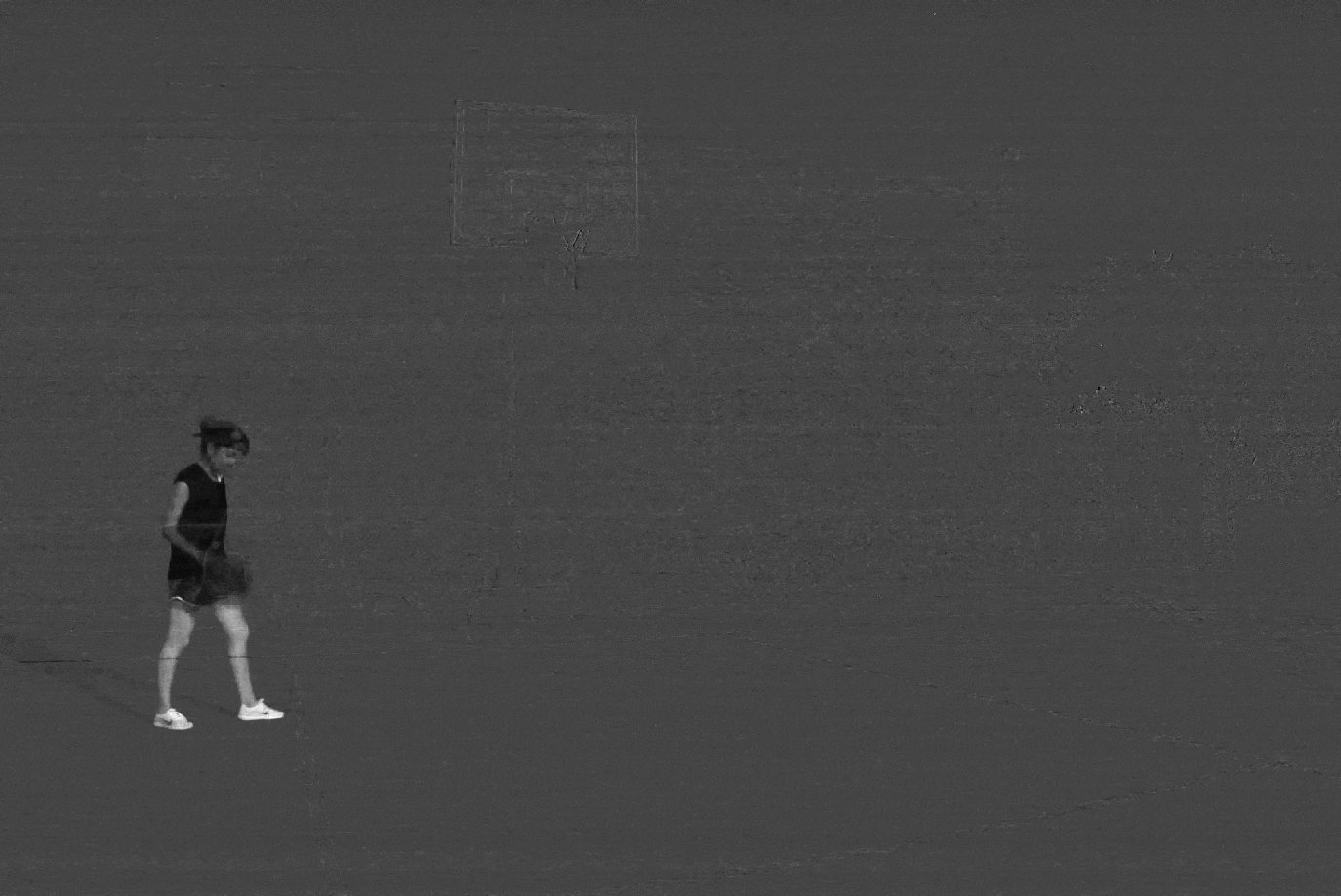