Last active
January 4, 2016 11:39
-
-
Save nojima/8616335 to your computer and use it in GitHub Desktop.
ロジスティック回帰
This file contains hidden or bidirectional Unicode text that may be interpreted or compiled differently than what appears below. To review, open the file in an editor that reveals hidden Unicode characters.
Learn more about bidirectional Unicode characters
合否 | 内申点 | 模試偏差値 | |
---|---|---|---|
0 | 3.6 | 60.1 | |
1 | 4.1 | 52.0 | |
0 | 3.7 | 62.5 | |
0 | 4.9 | 60.6 | |
1 | 4.4 | 54.1 | |
0 | 3.6 | 63.6 | |
1 | 4.9 | 68.0 | |
0 | 4.8 | 38.2 | |
1 | 4.1 | 59.5 | |
0 | 4.3 | 47.3 | |
1 | 4.6 | 61.1 | |
0 | 3.8 | 49.9 |
This file contains hidden or bidirectional Unicode text that may be interpreted or compiled differently than what appears below. To review, open the file in an editor that reveals hidden Unicode characters.
Learn more about bidirectional Unicode characters
#!/usr/bin/env python | |
from __future__ import print_function, unicode_literals | |
from argparse import ArgumentParser, FileType | |
import numpy as np | |
import scipy as sp | |
import matplotlib.pyplot as plt | |
def sigmoid(t): | |
return 1.0 / (1.0 + np.exp(-t)) | |
def gradient(model_w, data_t, data_x): | |
# parameter: | |
# model_w: d-dimentional vector | |
# data_t: n-dimentional vector | |
# data_x: (n, d)-dimentional matrix | |
# returns: | |
# d-dimentional gradient vector | |
return sum(data_x[i, :] * (sigmoid(np.dot(model_w, data_x[i, :])) - data_t[i]) | |
for i in xrange(len(data_t))) | |
def residual(model_w, data_t, data_x): | |
# parameter: | |
# model_w: d-dimentional vector | |
# data_t: n-dimentional vector | |
# data_x: (n, d)-dimentional matrix | |
# returns: | |
# scalar | |
r = 0 | |
for i in xrange(len(data_t)): | |
s = sigmoid(np.dot(model_w, data_x[i, :])) | |
r -= (1.0 - data_t[i]) * np.log(1.0 - s) + data_t[i] * np.log(s) | |
return r | |
def learn(training_file, skip, step, convit, maxit): | |
data = np.loadtxt(training_file, skiprows=skip, delimiter="\t") | |
data_n = len(data) | |
data_t = data[:, 0] | |
data_x = np.hstack((np.ones((data_n, 1)), data[:, 1:])) | |
d = data_x.shape[1] | |
# normalize | |
max_x = np.max(data_x, axis=0) | |
data_x /= max_x[np.newaxis, :] | |
model_w = sp.rand(d) | |
e = residual(model_w, data_t, data_x) | |
for i in xrange(maxit): | |
model_w -= step * gradient(model_w, data_t, data_x) | |
new_e = residual(model_w, data_t, data_x) | |
if np.abs(new_e - e) < convit: | |
break | |
e = new_e | |
# denormalize | |
data_x *= max_x[np.newaxis, :] | |
model_w /= max_x | |
return model_w, data_t, data_x | |
def visualize(model_w, data_t, data_x): | |
min_x = np.min(data_x, axis=0) | |
max_x = np.max(data_x, axis=0) | |
width = max_x - min_x | |
max_x += width * 0.05 | |
min_x -= width * 0.05 | |
x_range = np.arange(min_x[1], max_x[1], (max_x[1] - min_x[1]) / 100) | |
y_range = np.arange(min_x[2], max_x[2], (max_x[2] - min_x[2]) / 100) | |
z = sigmoid(model_w[0] + model_w[1] * x_range + model_w[2] * y_range[:, np.newaxis]) | |
plt.contourf(x_range, y_range, z, levels=np.arange(0.0, 1.0, 0.05)) | |
ok = data_t == 1 | |
ng = data_t == 0 | |
plt.plot(data_x[ok, 1], data_x[ok, 2], "bo") | |
plt.plot(data_x[ng, 1], data_x[ng, 2], "ro") | |
plt.show() | |
def main(): | |
parser = ArgumentParser() | |
parser.add_argument("training_file", | |
type=FileType("r"), metavar="TRAINING_FILE", | |
help="TSV file containing training data") | |
parser.add_argument("--skip", | |
type=int, metavar="LINES", default=0, | |
help="the number of lines to skip") | |
parser.add_argument("--step", | |
type=float, metavar="NUM", default=0.1) | |
parser.add_argument("--convit", | |
type=float, metavar="NUM", default=0.0001) | |
parser.add_argument("--maxit", | |
type=int, metavar="NUM", default=10000) | |
args = parser.parse_args() | |
model_w, data_t, data_x = learn(args.training_file, skip=args.skip, step=args.step, | |
convit=args.convit, maxit=args.maxit) | |
print(model_w) | |
visualize(model_w, data_t, data_x) | |
if __name__ == "__main__": | |
main() |
This file contains hidden or bidirectional Unicode text that may be interpreted or compiled differently than what appears below. To review, open the file in an editor that reveals hidden Unicode characters.
Learn more about bidirectional Unicode characters
$ logistic.py --skip 1 --convit 0.000001 --step 0.1 --maxit 100000 exam.tsv | |
[-13.14679925 1.80155595 0.09173275] |
Sign up for free
to join this conversation on GitHub.
Already have an account?
Sign in to comment
結果:
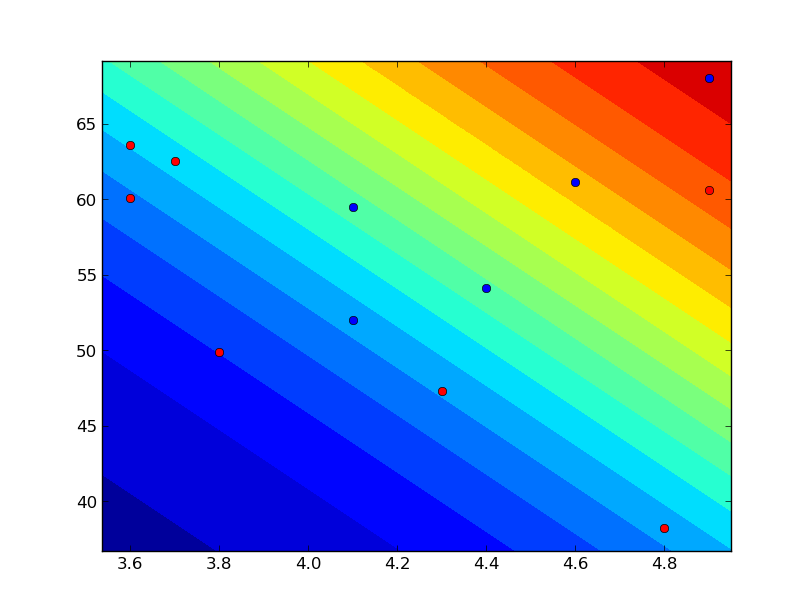