Last active
August 9, 2022 16:34
-
-
Save theorm/224f20af1b52216c969c98ddeebf116b to your computer and use it in GitHub Desktop.
Serve a fairseq summary model as an API
This file contains hidden or bidirectional Unicode text that may be interpreted or compiled differently than what appears below. To review, open the file in an editor that reveals hidden Unicode characters.
Learn more about bidirectional Unicode characters
FROM python:3.6.6-slim | |
WORKDIR /fia | |
RUN apt-get update | |
# the big one | |
RUN pip install torch | |
RUN apt-get install -y --no-install-recommends build-essential wget | |
COPY requirements.txt ./ | |
RUN pip install --no-cache-dir -r requirements.txt | |
RUN wget -O - https://github.com/microsoft/MASS/tarball/cda9f59 | tar xz | |
RUN mv microsoft-MASS-cda9f59 MASS | |
COPY fairseq-inference-api.py . |
This file contains hidden or bidirectional Unicode text that may be interpreted or compiled differently than what appears below. To review, open the file in an editor that reveals hidden Unicode characters.
Learn more about bidirectional Unicode characters
import re | |
from collections import namedtuple | |
import torch | |
from pytorch_transformers import BertTokenizer | |
from fairseq import checkpoint_utils, options, tasks, utils | |
from fairseq.data import encoders | |
bert_tokenizer = BertTokenizer.from_pretrained('bert-base-uncased') | |
def tokenize_with_bert(sentence): | |
return ' '.join(bert_tokenizer.tokenize(sentence)) | |
def detokenize_with_bert(sentence): | |
return re.sub(r' ##', '', sentence) | |
Batch = namedtuple('Batch', 'ids src_tokens src_lengths') | |
def buffered_read(lines_of_text, buffer_size): | |
for line in lines_of_text: | |
yield line | |
def make_batches(lines, args, task, max_positions, encode_fn): | |
tokens = [ | |
task.source_dictionary.encode_line( | |
encode_fn(src_str), add_if_not_exist=False | |
).long() | |
for src_str in lines | |
] | |
lengths = torch.LongTensor([t.numel() for t in tokens]) | |
itr = task.get_batch_iterator( | |
dataset=task.build_dataset_for_inference(tokens, lengths), | |
max_tokens=args.max_tokens, | |
max_sentences=args.max_sentences, | |
max_positions=max_positions, | |
).next_epoch_itr(shuffle=False) | |
for batch in itr: | |
yield Batch( | |
ids=batch['id'], | |
src_tokens=batch['net_input']['src_tokens'], src_lengths=batch['net_input']['src_lengths'], | |
) | |
class FairseqRunner: | |
def __init__(self, input_args = None): | |
parser = options.get_generation_parser(interactive=True) | |
args = options.parse_args_and_arch(parser, input_args) | |
utils.import_user_module(args) | |
if args.buffer_size < 1: | |
args.buffer_size = 1 | |
if args.max_tokens is None and args.max_sentences is None: | |
args.max_sentences = 1 | |
assert not args.sampling or args.nbest == args.beam, \ | |
'--sampling requires --nbest to be equal to --beam' | |
assert not args.max_sentences or args.max_sentences <= args.buffer_size, \ | |
'--max-sentences/--batch-size cannot be larger than --buffer-size' | |
# print(args) | |
use_cuda = torch.cuda.is_available() and not args.cpu | |
# Setup task, e.g., translation | |
task = tasks.setup_task(args) | |
# Load ensemble | |
print('| loading model(s) from {}'.format(args.path)) | |
models, _model_args = checkpoint_utils.load_model_ensemble( | |
args.path.split(':'), | |
arg_overrides=eval(args.model_overrides), | |
task=task, | |
) | |
# Set dictionaries | |
src_dict = task.source_dictionary | |
tgt_dict = task.target_dictionary | |
# Optimize ensemble for generation | |
for model in models: | |
model.make_generation_fast_( | |
beamable_mm_beam_size=None if args.no_beamable_mm else args.beam, | |
need_attn=args.print_alignment, | |
) | |
if args.fp16: | |
model.half() | |
if use_cuda: | |
model.cuda() | |
# Initialize generator | |
generator = task.build_generator(args) | |
# Handle tokenization and BPE | |
tokenizer = encoders.build_tokenizer(args) | |
bpe = encoders.build_bpe(args) | |
# Load alignment dictionary for unknown word replacement | |
# (None if no unknown word replacement, empty if no path to align dictionary) | |
align_dict = utils.load_align_dict(args.replace_unk) | |
max_positions = utils.resolve_max_positions( | |
task.max_positions(), | |
*[model.max_positions() for model in models] | |
) | |
if args.buffer_size > 1: | |
print('| Sentence buffer size:', args.buffer_size) | |
self.context = { | |
'bpe': bpe, | |
'tokenizer': tokenizer, | |
'args': args, | |
'task': task, | |
'max_positions': max_positions, | |
'use_cuda': use_cuda, | |
'generator': generator, | |
'models': models, | |
'src_dict': src_dict, | |
'tgt_dict': tgt_dict, | |
'align_dict': align_dict, | |
} | |
def infer(self, lines_of_text): | |
context = self.context | |
bpe = context['bpe'] | |
tokenizer = context['tokenizer'] | |
args = context['args'] | |
task = context['task'] | |
max_positions = context['max_positions'] | |
use_cuda = context['use_cuda'] | |
generator = context['generator'] | |
models = context['models'] | |
src_dict = context['src_dict'] | |
tgt_dict = context['tgt_dict'] | |
align_dict = context['align_dict'] | |
def encode_fn(x): | |
x = tokenize_with_bert(x) | |
if tokenizer is not None: | |
x = tokenizer.encode(x) | |
if bpe is not None: | |
x = bpe.encode(x) | |
return x | |
def decode_fn(x): | |
if bpe is not None: | |
x = bpe.decode(x) | |
if tokenizer is not None: | |
x = tokenizer.decode(x) | |
x = detokenize_with_bert(x) | |
return x | |
start_id = 0 | |
# for inputs in buffered_read(args.input, args.buffer_size): | |
for inputs in [lines_of_text]: | |
results = [] | |
for batch in make_batches(inputs, args, task, max_positions, encode_fn): | |
src_tokens = batch.src_tokens | |
src_lengths = batch.src_lengths | |
if use_cuda: | |
src_tokens = src_tokens.cuda() | |
src_lengths = src_lengths.cuda() | |
sample = { | |
'net_input': { | |
'src_tokens': src_tokens, | |
'src_lengths': src_lengths, | |
}, | |
} | |
translations = task.inference_step(generator, models, sample) | |
for i, (id, hypos) in enumerate(zip(batch.ids.tolist(), translations)): | |
src_tokens_i = utils.strip_pad(src_tokens[i], tgt_dict.pad()) | |
results.append((start_id + id, src_tokens_i, hypos)) | |
# sort output to match input order | |
for id, src_tokens, hypos in sorted(results, key=lambda x: x[0]): | |
if src_dict is not None: | |
src_str = src_dict.string(src_tokens, args.remove_bpe) | |
# print('S-{}\t{}'.format(id, src_str)) | |
# Process top predictions | |
for hypo in hypos[:min(len(hypos), args.nbest)]: | |
hypo_tokens, hypo_str, alignment = utils.post_process_prediction( | |
hypo_tokens=hypo['tokens'].int().cpu(), | |
src_str=src_str, | |
alignment=hypo['alignment'], | |
align_dict=align_dict, | |
tgt_dict=tgt_dict, | |
remove_bpe=args.remove_bpe, | |
) | |
hypo_str = decode_fn(hypo_str) | |
yield (hypo_str, hypo, hypo_tokens) | |
# print('H-{}\t{}\t{}'.format(id, hypo['score'], hypo_str)) | |
# print('P-{}\t{}'.format( | |
# id, | |
# ' '.join(map(lambda x: '{:.4f}'.format(x), hypo['positional_scores'].tolist())) | |
# )) | |
# if args.print_alignment: | |
# alignment_str = " ".join(["{}-{}".format(src, tgt) for src, tgt in alignment]) | |
# print('A-{}\t{}'.format( | |
# id, | |
# alignment_str | |
# )) | |
# update running id counter | |
start_id += len(inputs) | |
if __name__ == '__main__': | |
from flask import Flask, escape, request | |
app = Flask(__name__) | |
runner = FairseqRunner() | |
@app.route('/', methods=['POST']) | |
def hello(): | |
if request.json is None or 'text' not in request.json: | |
return { 'error': '"text" field in JSON payload is required'}, 400 | |
text = request.json.get('text') | |
if not isinstance(text, list): | |
return { 'error': '"text" is expected to be a list of texts pieces'}, 400 | |
summary = [s for s, hypo, tokens in runner.infer(text)] | |
return { 'ok': True, 'text': text, 'summary': summary } | |
app.run('0.0.0.0', 3000) |
This file contains hidden or bidirectional Unicode text that may be interpreted or compiled differently than what appears below. To review, open the file in an editor that reveals hidden Unicode characters.
Learn more about bidirectional Unicode characters
build: | |
docker build -t theorm/fairseq-inference-api-wip . | |
run: | |
docker run \ | |
--rm -it \ | |
-p 3000:3000 \ | |
--name fairseq-inference-api-wip \ | |
-v $(PWD)/../../checkpoints:/checkpoints \ | |
-v $(PWD)/../../dicts:/dicts \ | |
theorm/fairseq-inference-api-wip \ | |
python fairseq-inference-api.py \ | |
--user-dir ./MASS/MASS-summarization/mass \ | |
--path /checkpoints/checkpoint_best.pt \ | |
--beam 5 \ | |
--no-repeat-ngram-size 3 \ | |
--lenpen 1.0 \ | |
--task translation_mass \ | |
--source-lang src --target-lang tgt \ | |
/dicts | |
push: | |
docker push theorm/fairseq-inference-api-wip |
This file contains hidden or bidirectional Unicode text that may be interpreted or compiled differently than what appears below. To review, open the file in an editor that reveals hidden Unicode characters.
Learn more about bidirectional Unicode characters
idna==2.8 | |
pytorch-transformers==1.2.0 | |
# torch==1.3.0 | |
flask==1.1.1 | |
fairseq==0.8.0 |
Sign up for free
to join this conversation on GitHub.
Already have an account?
Sign in to comment
Hello, I remove flask part from fairseq-inference-api.py, and "text" is initialized to [text1], when I run: python fairseq-inference-api.py
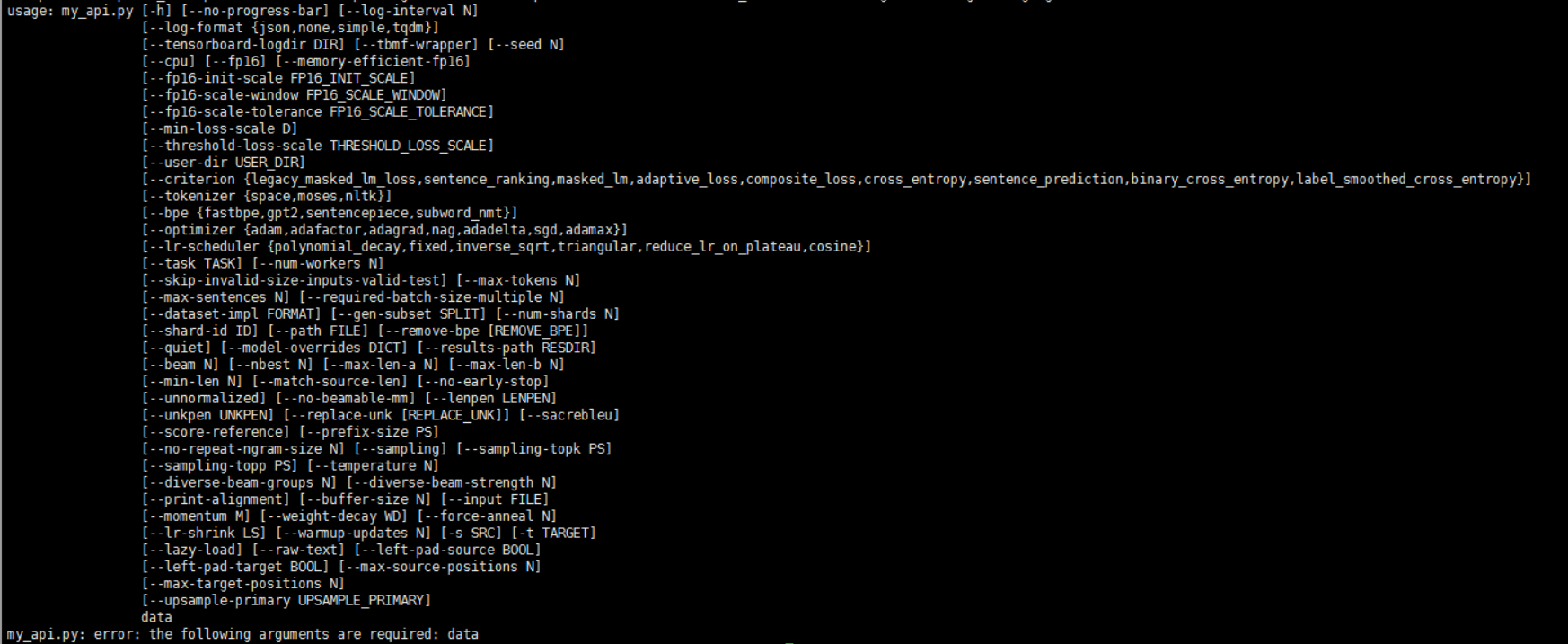
--user-dir ./MASS/MASS-summarization/mass
--path /checkpoints/checkpoint_best.pt
--beam 5
--no-repeat-ngram-size 3
--lenpen 1.0
--task translation_mass
--source-lang src --target-lang tgt
I encountered the following error:
Do you konw why?
Thank you.