Created
November 22, 2017 14:35
-
-
Save FloWuenne/b4d1729b5ec2ceecfb4ce532e0fd8d67 to your computer and use it in GitHub Desktop.
Plot PCA for ethnicity from any given VCF file combined with 1000 genomes data
This file contains hidden or bidirectional Unicode text that may be interpreted or compiled differently than what appears below. To review, open the file in an editor that reveals hidden Unicode characters.
Learn more about bidirectional Unicode characters
## Plot PCA for ethnicity of a given VCF file | |
## Import publication theme | |
## A nice R ggplot theme that I found online. Original can be found here: | |
## https://rpubs.com/Koundy/71792 | |
theme_Publication <- function(base_size=14, base_family="helvetica") { | |
library(grid) | |
library(ggthemes) | |
(theme_foundation(base_size=base_size, base_family=base_family) | |
+ theme(plot.title = element_text(face = "bold", | |
size = rel(1.2), hjust = 0.5), | |
text = element_text(), | |
panel.background = element_rect(colour = NA), | |
plot.background = element_rect(colour = NA), | |
panel.border = element_rect(colour = NA), | |
axis.title = element_text(face = "bold",size = rel(1)), | |
axis.title.y = element_text(angle=90,vjust =2), | |
axis.title.x = element_text(vjust = -0.2), | |
axis.text = element_text(), | |
axis.line = element_line(colour="black"), | |
axis.ticks = element_line(), | |
panel.grid.major = element_line(colour="#f0f0f0"), | |
panel.grid.minor = element_blank(), | |
legend.key = element_rect(colour = NA), | |
legend.position = "bottom", | |
legend.direction = "horizontal", | |
legend.key.size= unit(0.2, "cm"), | |
legend.margin = unit(0, "cm"), | |
legend.title = element_text(face="italic"), | |
plot.margin=unit(c(10,5,5,5),"mm"), | |
strip.background=element_rect(colour="#f0f0f0",fill="#f0f0f0"), | |
strip.text = element_text(face="bold") | |
)) | |
} | |
scale_fill_Publication <- function(...){ | |
library(scales) | |
discrete_scale("fill","Publication",manual_pal(values = c("#386cb0","#fdb462","#7fc97f","#ef3b2c","#662506","#a6cee3","#fb9a99","#984ea3","#ffff33")), ...) | |
} | |
scale_colour_Publication <- function(...){ | |
library(scales) | |
discrete_scale("colour","Publication",manual_pal(values = c("#386cb0","#fdb462","#7fc97f","#ef3b2c","#662506","#a6cee3","#fb9a99","#984ea3","#ffff33")), ...) | |
} | |
## Import libraries | |
library(gdsfmt) | |
library(SNPRelate) | |
library(ggplot2) | |
library(ggdendro) | |
library(RColorBrewer) | |
library(plyr) | |
library(dplyr) | |
## Set working directory | |
## Set color palette for populations | |
color_palette <- c("#912CEE","#f40000","#4DBD33","#4885ed","#ffc300","#2b2b28") | |
## Specify your VCF file | |
vcf.fn <- "my.vcf" | |
# Transform the VCF file into gds format | |
snpgdsVCF2GDS(vcf.fn, "Variants_1000G_exomes.gds", method="biallelic.only") | |
## Data Analysis | |
## open the newly created gds file | |
genofile <- snpgdsOpen('Variants_1000G_exomes.gds') | |
## LD based SNP pruning | |
set.seed(1000) | |
snpset <- snpgdsLDpruning(genofile, ld.threshold=0.2) | |
snpset.id <- unlist(snpset) | |
## Perform PCA on the SNP data | |
pca <- snpgdsPCA(genofile, snp.id=snpset.id, num.thread=4) | |
pc.percent <- pca$varprop*100 | |
head(round(pc.percent, 2)) | |
### Plotting | |
### Plot results from PCA analysis of variant data | |
tab12 <- data.frame(sample.id = pca$sample.id, | |
EV1 = pca$eigenvect[,1], # the first eigenvector | |
EV2 = pca$eigenvect[,2], # the second eigenvector | |
stringsAsFactors = FALSE) | |
tab34 <- data.frame(sample.id = pca$sample.id, | |
EV3 = pca$eigenvect[,3], # the first eigenvector | |
EV4 = pca$eigenvect[,4], # the second eigenvector | |
stringsAsFactors = FALSE) | |
plot(tab34$EV2, tab34$EV1, xlab="eigenvector 3", ylab="eigenvector 4") | |
## Assign ethnicity description from 1000G file | |
sample.id <- read.gdsn(index.gdsn(genofile, "sample.id")) | |
populations <- read.table("All_samples_populations.tab",header=T) | |
tab <- data.frame(sample.id = pca$sample.id, | |
EV1 = pca$eigenvect[,1], # the first eigenvector | |
EV2 = pca$eigenvect[,2], # the second eigenvector | |
stringsAsFactors = FALSE) | |
colnames(tab) <- c("sample.id","EV1","EV2") | |
PCA_allVariants2 <- ggplot(tab,aes(x=EV1,y=EV2)) + | |
geom_point(size=2) + | |
scale_x_continuous("PC1") + | |
scale_y_continuous("PC2") + | |
scale_colour_manual(values=color_palette[1:length(unique(tab$pop))]) + | |
theme_Publication() | |
PCA_allVariants2 | |
ggsave(file="Variants_PCA_pointsize2.svg",plot=PCA_allVariants2) | |
ggsave(file="Variants_PCA_pointsize1.svg",plot=PCA_allVariants1) |
Sign up for free
to join this conversation on GitHub.
Already have an account?
Sign in to comment
Hello Florian
I removed the chrM from my data file then change the chr1 chr2 chr3 into 1 2 3 as per the fasta file to make the vcf file and the fasta file have the same.I am using the GRCh37 / hg19 reference genome.
first i was gettinh chrM not found then i removed the chrM and i get the chr1 not found error which explains the difference between my fasta and my vcf file so i used emeditor an editor to change my vcf file (as other methods were not preserving the header).
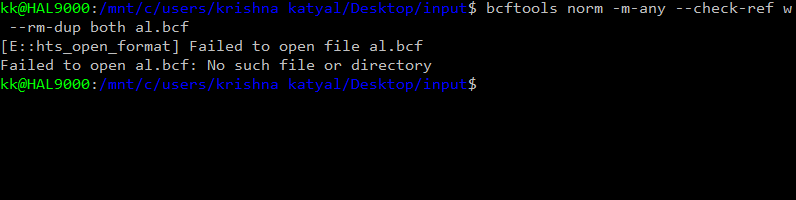
now chrM is gone the annotation of fasta and vcf is same but still i get the error
code :
bcftools norm -m-any --check-ref w -f human_g1k_v37.fasta \
data.vcf | \
bcftools annotate -x ID -I +'%CHROM:%POS:%REF:%ALT' | \
bcftools norm -Ob --rm-dup both \
> data.bcf ;
`
I am giving to the orginal data(it is single sample) which will make it is easier for check the annotation error
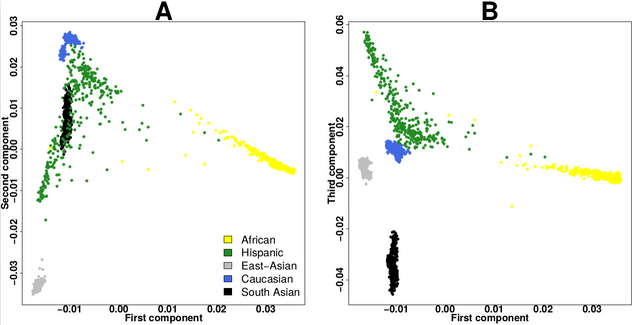
Link to my data
I think the plot should look like this(like the plot in the biostar tutorial)
Thanks alot for helping and keef safe !
i wish for your best health