Last active
September 19, 2020 00:09
-
-
Save torfjelde/ffb61275165212520c055978474e663d to your computer and use it in GitHub Desktop.
An example of how to use TensorboardLogging.jl to log certain statistics during sampling in Turing.jl
This file contains bidirectional Unicode text that may be interpreted or compiled differently than what appears below. To review, open the file in an editor that reveals hidden Unicode characters.
Learn more about bidirectional Unicode characters
using Turing | |
using TensorBoardLogger, Logging | |
using OnlineStats # used to compute different statistics on-the-fly | |
using StatsBase # Provides us with the `Histogram` which is supported by `TensorBoardLogger.jl` | |
using LinearAlgebra | |
using DataStructures # will use a `CircularBuffer` to only keep track of some `n` last samples | |
struct TBCallback | |
logger::TBLogger | |
end | |
function TBCallback(dir::String) | |
# Set up the logger | |
lg = TBLogger(dir, min_level=Logging.Info; step_increment=0) | |
return TBCallback(lg) | |
end | |
make_estimator(cb::TBCallback, num_bins::Int) = OnlineStats.Series( | |
Mean(), # Online estimator for the mean | |
Variance(), # Online estimator for the variance | |
KHist(num_bins) # Online estimator of a histogram with `100` bins | |
) | |
make_buffer(cb::TBCallback, window::Int) = CircularBuffer{Float64}(window) | |
# Convenience method for taking a histogram with centers to edges | |
function centers_to_edges(centers) | |
# Find the midpoint between the nearby centers. | |
intermediate = map(2:length(centers)) do i | |
# Pick the left mid-point | |
(centers[i] + centers[i - 1]) / 2 | |
end | |
# Left-most point | |
Δ_l = (centers[2] - centers[1]) / 2 | |
leftmost = centers[1] - Δ_l | |
# Right-most point | |
Δ_r = (centers[end] - centers[end - 1]) / 2 | |
rightmost = centers[end] + Δ_r | |
return vcat([leftmost], intermediate, [rightmost]) | |
end | |
function make_callback( | |
cb::TBCallback, | |
spl::Turing.InferenceAlgorithm, # used to extract sampler-specific parameters in the future | |
num_samples::Int; | |
num_bins::Int = 100, | |
window::Int = min(num_samples, 1_000), | |
window_num_bins::Int = 50 | |
) | |
lg = cb.logger | |
# Lookups | |
estimators = Dict{String, typeof(make_estimator(cb, num_bins))}() | |
buffers = Dict{String, typeof(make_buffer(cb, window))}() | |
return function callback(rng, model, sampler, transition, iteration) | |
with_logger(lg) do | |
for (vals, ks) in values(transition.θ) | |
for (k, val) in zip(ks, vals) | |
if !haskey(estimators, k) | |
estimators[k] = make_estimator(cb, num_bins) | |
end | |
est = estimators[k] | |
if !haskey(buffers, k) | |
buffers[k] = make_buffer(cb, window) | |
end | |
buffer = buffers[k] | |
# Log the raw value | |
@info k val | |
# Update buffer and estimator | |
push!(buffer, val) | |
fit!(est, val) | |
mean, variance, hist_raw = value(est) | |
# Need some iterations before we start showing the stats | |
if iteration > 10 | |
# Convert `OnlineStats.KHist` to `StatsBase.Histogram` | |
edges = centers_to_edges(hist_raw.centers) | |
cnts = hist_raw.counts ./ sum(hist_raw.counts) | |
hist = Histogram(edges, cnts, :left, true) | |
# `normalize` ensures the `Histogram` sums to 1 | |
hist_window = normalize(fit( | |
Histogram, collect(buffer); | |
nbins = window_num_bins | |
), mode = :density) | |
@info "$k" mean | |
@info "$k" var | |
@info "$k" hist | |
@info "$k" hist_window | |
# Because the `Distribution` and `Histogram` functionality in | |
# TB is quite crude, we additionally log "later" values to provide | |
# a slightly more useful view of the later samples in the chain. | |
# TODO: make this, say, 25% of the total number of iterations | |
if iteration > 0.25 * num_samples | |
@info "$k/late" mean | |
@info "$k/late" var | |
@info "$k/late" hist | |
@info "$k/late" hist_window | |
end | |
end | |
end | |
end | |
# Increment the step | |
@info "log joint prob" DynamicPPL.getlogp(transition) log_step_increment=1 | |
# TODO: log additional sampler stats, e.g. rejection rate, numerical_errors | |
end | |
end | |
end | |
############### | |
### Example ### | |
############### | |
@model function demo(x) | |
s ~ InverseGamma(2, 3) | |
m ~ Normal(0, √s) | |
for i in eachindex(x) | |
x[i] ~ Normal(m, √s) | |
end | |
end | |
xs = randn(100) .+ 1; | |
model = demo(xs); | |
# Number of MCMC samples/steps | |
num_samples = 50_000 | |
# Sampling algorithm to use | |
alg = NUTS(0.65) | |
# Create the callback | |
callback = make_callback(TBCallback("tensorboard_logs/run"), alg, num_samples) | |
# Sample | |
sample(model, alg, num_samples; callback = callback) |
Sign up for free
to join this conversation on GitHub.
Already have an account?
Sign in to comment
Some pictures of what it looks like during sampling:
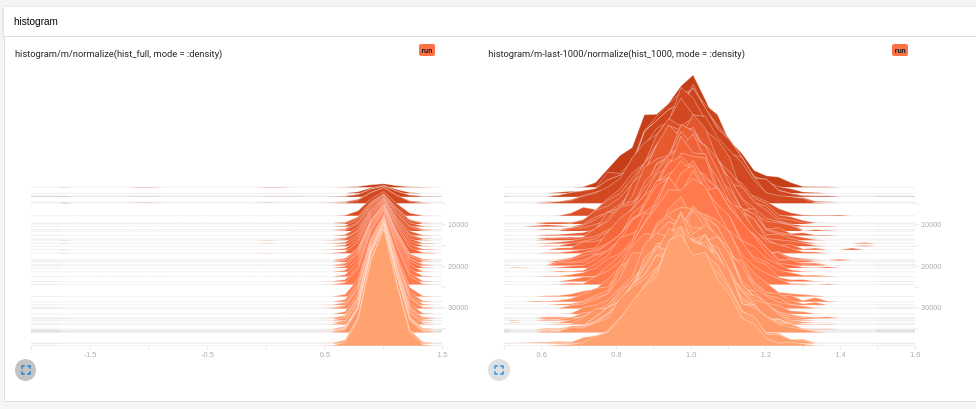
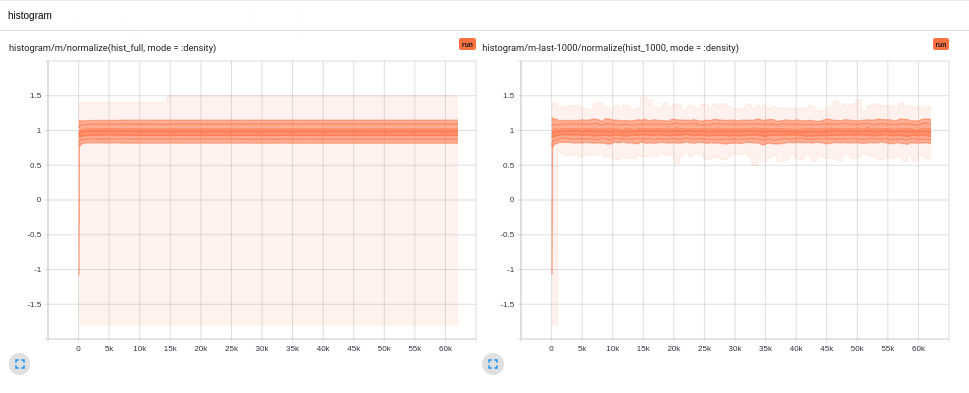
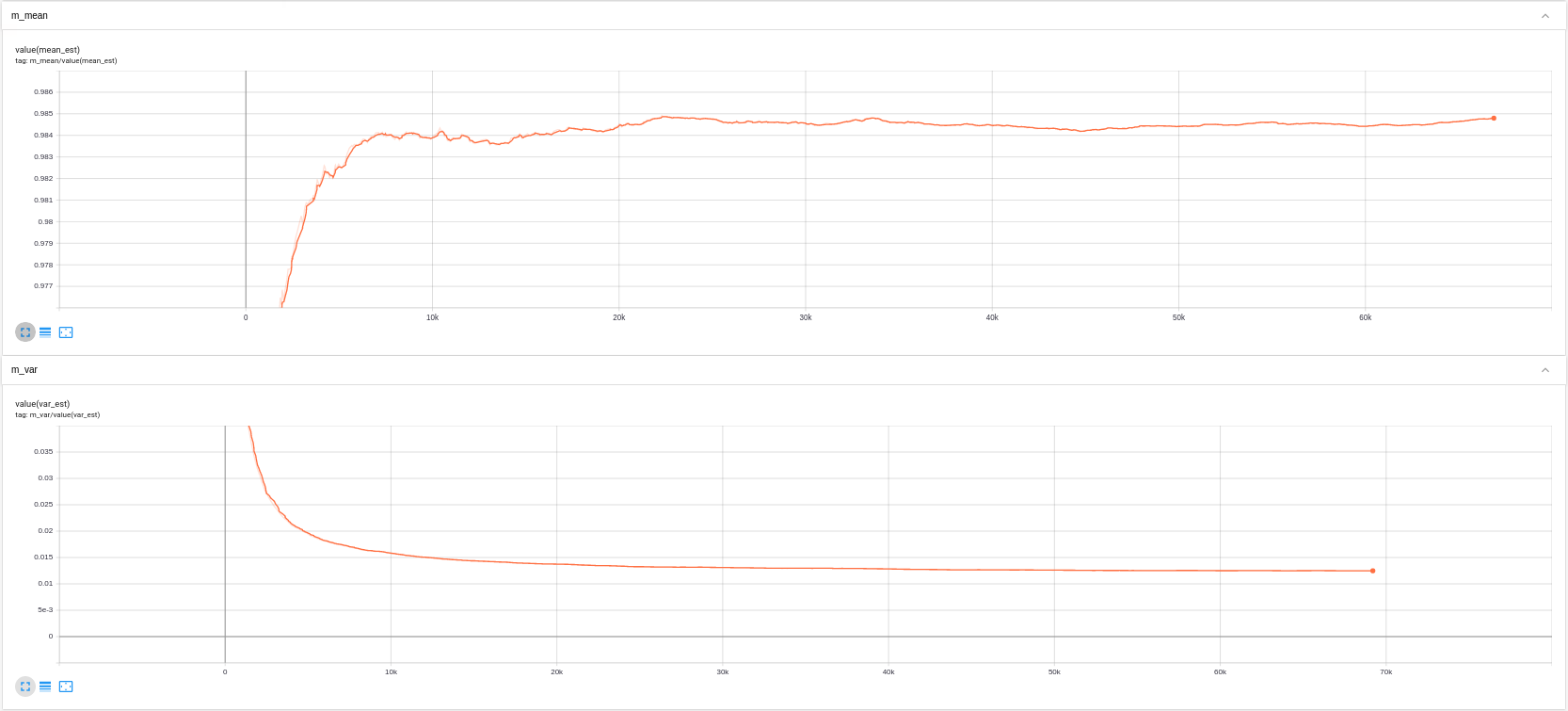